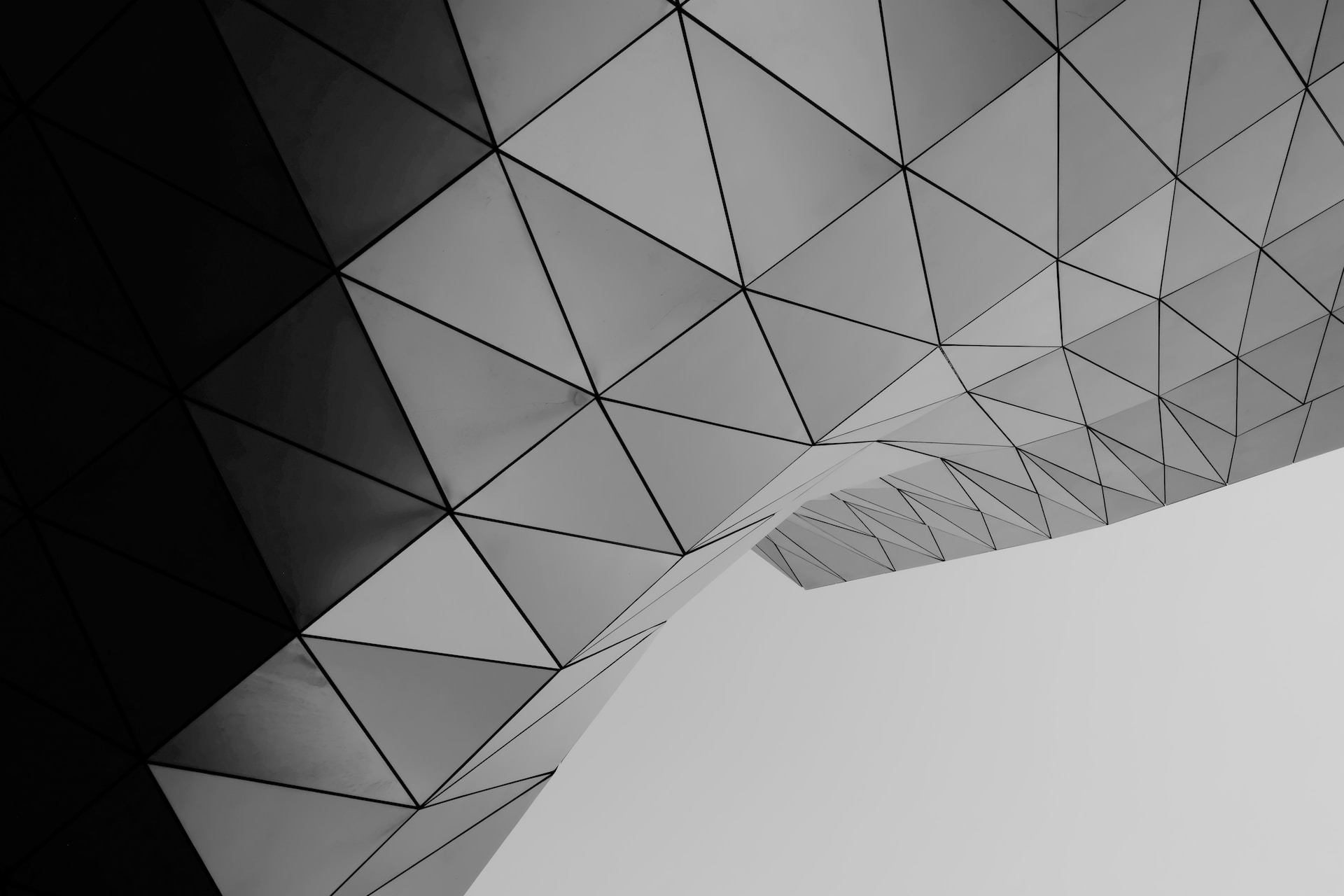
AI regulation in the world is still uncertain, and the best way to prepare for it is by applying AI ethics principles. They are value-based and applied on a voluntary basis. However, many upcoming regulations are drawn from them, which renders the adherence to good ethics even more so compelling.
Several organizations have issued their own set of AI ethics principles, such as the OECD, the UNESCO, or the European Union, among many others. In this article, I aim to show the most commonly shared AI ethics principles.
The Difference Between AI Ethics Principles and AI Regulation
Generally, ethics revolves around moral principles guiding behavior based on what’s considered right or wrong, broader in scope and flexible, relying on voluntary adherence. Regulations, on the other hand, are specific, legally binding rules established by authorities, enforced with clear guidelines and consequences, focusing on controlling and governing particular activities or industries. While ethics inform values, regulations are concrete laws with mandatory compliance.
In the field of artificial intelligence, regulation takes the form of binding requirements, often addressed at the providers of AI solutions, and enforced by the competent authorities. Taking the example of the AI Act in the EU, this regulation adopts a risk-based approach and classifies AI systems based on the risk they present for society, ranging from non risky (eg a spam filter or video games) to unacceptable (such as real-time facial recognition in public spaces or social credit systems). Providers of such systems will face different obligations, but privacy is not part of the AI Act, and is handled instead by GDPR, with its own sets of rules.
AI Ethics Principles, in a nutshell, are more comprehensive and intend to cover all the ways in which artificial intelligence should be built and used responsibly. They are applied voluntarily and not enforced by a state authority.
The 7 AI Ethics Principles
-
Fairness and Bias Mitigation
- Definition: Ensuring AI systems don’t discriminate or reinforce biases against particular individuals or groups.
- Example: In hiring processes, AI algorithms used to screen resumes must be designed to avoid biases based on gender, race, or ethnicity. The system should ensure equal consideration for all applicants.
- Potential solution: Regularly audit algorithms for biases, use diverse and representative datasets, employ techniques like adversarial debiasing, and implement fairness-aware machine learning algorithms.
-
Transparency and Explainability
- Definition: Making AI systems understandable by providing explanations for their decisions or actions.
- Example: AI-powered loan approval systems should provide clear explanations for why a loan application was accepted or rejected. This transparency helps applicants understand the decision-making process.
- Potential Solution: Develop AI models that generate explanations for their decisions (e.g., interpretable machine learning models), provide user-friendly interfaces to display AI-generated outcomes, and use tools for model interpretability.
-
Accountability and Responsibility
- Definition: Establishing data governance and mechanisms to assign responsibility when AI systems cause harm or make incorrect decisions.
- Example: Establishing clear protocols to determine who is responsible when an autonomous vehicle is involved in an accident. This includes defining whether it’s the manufacturer, the software developer, or the vehicle owner.
- Potential Solution: Establish clear guidelines for AI system development, define protocols for assigning responsibility in case of system failures, and institute policies that hold organizations accountable for their AI systems’ behavior.
-
Privacy and Data Rights
- Definition: Safeguarding user data and ensuring that AI systems handle and process information in a manner that respects privacy rights and data protection regulations. Most GDPR compliant organizations will be well equipped for this one.
- Example: Implementing robust encryption and access controls to protect sensitive medical data in AI systems used for healthcare, ensuring patient privacy is maintained.
- Potential Solution: Perform a robust Data Protection Impact Assessment (DPIA) on the AI solution. Ensure you know what happens to the personal data processed as part of the AI system (even more so if they are training the AI). Crucially, users should be adequately trained to use the AI in the way it’s intended to be.
-
Safety and Reliability
- Definition: Developing AI systems that are safe, reliable, and free from unintended consequences or risks, including attacks.
- Example: Testing and validating AI systems extensively before deploying them in critical domains like healthcare diagnosis or autonomous vehicles, ensuring they perform reliably and safely.
- Potential Solution: Conduct rigorous testing, validation, and simulation of AI systems in various scenarios, adopt fail-safe mechanisms like kill switches, and continuously monitor AI systems for unexpected behavior.
-
Societal Impact and Benefit
- Definition: Considering the broader impact of AI on society, aiming for positive contributions to societal well-being.
- Example: Using AI to improve accessibility, such as developing systems that assist individuals with disabilities, thereby promoting inclusivity and societal benefit.
- Potential Solution: Conduct thorough impact assessments prior to deploying AI systems, collaborate with diverse stakeholders, and invest in projects that address societal needs, ensuring AI benefits all segments of society.
-
Human Control and Autonomy
- Definition: Ensuring that humans remain in control of AI systems and that these systems respect human autonomy, dignity, and decision-making authority.
- Example: Designing AI systems in collaboration with humans, allowing human intervention or oversight when crucial decisions are made, ensuring ultimate control remains with humans.
- Potential Solution: Incorporate human-in-the-loop systems, allowing human oversight or intervention when critical decisions are made, and design AI systems that augment human capabilities rather than replacing them entirely.
Follow AI Ethics Principles Like a Guiding Beacon
In the ever-evolving realm of AI regulation, following AI ethics principles stands as a guiding beacon. These principles, akin to our moral compass, shape the responsible development of AI. They are the blueprint from which future regulations emerge. While regulations offer clear rules, ethics provide a broader, adaptable framework. Embracing these principles not only fosters ethical AI but also aligns with evolving regulations, ensuring AI that respects our values and uplifts society.